Multi-omics
Uncovering mechanisms of diseases and making medicine personalized
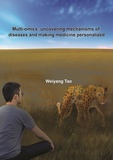
Tao, Weiyang
- Promoter:
- Prof.dr T.R.D.J. (Tim) Radstake
- Co-promoter:
- Dr A. (Aridaman) Pandit
- Research group:
- Radstake
- Date:
- March 30, 2021
- Time:
- 12:45 h
Summary
Multi-omics, meaning “multiple omics”, became accessible because of the revolution in high-throughput technologies. Omics in biology is a general term for analyzing biological information objects. A molecular term followed by the suffix “omics” implies a comprehensive, or global assessment of that set of molecules, such as genomics, epigenomics, transcriptomics, proteomics, phosphoproteomics, and metabolomics. Multi-omics approaches have been integrated and applied by many studies to a wide range of biological problems, including disease mechanism revealing, biomarker identification, and patient classification. The multi-omics involved in this thesis are epigenomics (DNA methylomics), transcriptomics, and proteomics. This thesis divides into two major parts, aiming at using multi-omics to provide a better understanding of autoimmune diseases, including systemic sclerosis (SSc), psoriatic arthritis (PsA), psoriasis (Pso), Ankylosing spondylitis (AS), and RA, even to depict a paradigm to study other diseases in general, ultimately facilitating personalized medicine. The first part focuses on using multi-omics to reveal disease mechanisms from a perspective of studying cytokine stimulation of cells. The second part mainly describes the (multi-)omic commonality and the distinction between patients with different autoimmune diseases or with different subtypes of the same disease, i.e., rheumatoid arthritis.
In the first part of the thesis, we first conducted a pilot study by using DNA methylomics and transcriptomics to investigate how the chemokine CXCL4 altered dendritic cell (DC) functions on molecular levels at the last day of monocyte-derived DC (moDC) differentiation. In Chapter 2, we found that CXCL4 suppressed tolerogenic DC gene signatures, such as IL10, and induced immunogenic DC gene signatures, such as CD86. Further analysis revealed that the perturbance of tolerogenic and immunogenic DC gene signatures was correlated with three genes (C1QA, C1QB, and C1QC) of a component (C1q) in the complement pathway, while the DNA methylation of C1q genes was negatively correlated with their gene expression. These findings indicate that CXCL4 might be involved in the dysregulation of DCs maturation via C1q, resulting in abnormality in immune homeostasis, which is a potential mechanism contributing to autoimmune diseases, such as SSc.
In Chapter 3, the moDC differentiation stimulated with or without CXCL4 was extensively studied by using DNA methylomics and transcriptomics. We demonstrated that CXCL4 dramatically altered the trajectory of monocyte differentiation, inducing a novel pro-inflammatory and pro-fibrotic phenotype. By establishing and using the RegEnrich pipeline, we predicted that this phenotype was mediated via key transcriptional regulators including CIITA. Importantly, these pro-inflammatory cells directly trigger a fibrotic cascade by producing extracellular matrix molecules and inducing myofibroblast differentiation. Inhibition of CIITA mimicked CXCL4 in inducing a pro-inflammatory and pro-fibrotic phenotype, validating the relevance of the gene regulatory network. Our study unveils that CXCL4 acts as a key secreted factor driving innate immune training and forming the long-sought link between inflammation and fibrosis, which might be involved in SSc pathogenesis.
In Chapter 4, we standardized the RegEnrich pipeline as an R/Bioconductor package, which outperformed a package with a similar function called VIPER in ranking the key regulators in gene silencing datasets. By applying RegEnrich to gene expression datasets of in vitro interferon-stimulation studies, we found that not only IRF and STAT transcription factor families played an important role in cells responding to IFN, but also several ETS transcription factor family members, such as ELF1 and ETV7, were highly associated with IFN stimulations. These findings mean that RegEnrich can accurately identify, in a data-driven manner, key gene regulators from the cells under different biological states, which can be valuable in mechanistically studying cell differentiation, cell response on drug stimulation, and diseases development. Taking together, the first part of this thesis reveals a potential molecular mechanism related to CXCL4 and DCs in SSc and ends up with a new tool for revealing future disease mechanisms.
In the second part of the thesis, we focused on using omic tools to compare patients who were classified based on classical disease definition or based on the responsiveness to a certain treatment. In Chapter 5, we used Olink to assess serum proteomics of healthy controls (HC) and patients with PsA, Pso, and AS, which were classified according to the clinical symptoms. We found 68 differentially expressed proteins (DEPs) in PsA as compared with HC. Of those DEPs, 48 proteins were also dysregulated in Pso and/or AS. However, there were no DEPs when comparing PsA with Pso directly. Unsupervised machine learning methods revealed that HC clustered distinctly from all patients and that PsA and Pso grouped together. These results suggest PsA and Pso patients had very similar serum proteomic signatures, supporting the concept of a single psoriatic spectrum of disease.
In Chapter 6, we performed transcriptomic and/or DNA methylomic profiling on peripheral blood mononuclear cells (PBMCs), monocytes, and CD4+ T cells, from 80 patients with RA prior initiation of TNF inhibitor (TNFi) treatments (i.e., ADA or ETN). The patients were classified into responders and non-responders to either ADA or ETN, according to the EULAR criteria. The results showed that transcriptional signatures associated ADA and ETN responsiveness were divergent in all cell subsets. Only the genes upregulated in CD4+ T cells of ADA responders, compared to non-responders, were enriched in the TNF signaling pathway. Differentially methylated positions (DMPs) of responders to ETN but not to ADA were majorly hypermethylated compared to the corresponding non-responders. We then built a supervised classification model using each dataset able to accurately predict patients’ response to ADA and ETN, which was validated by a follow-up study. Thus, the second part of this thesis provides a better understanding of the similarity in patients with Pso and PsA, and the distinction in RA patients who have different responses to TNFi therapy.
Altogether, this thesis provides not only the potential mechanisms of CXCL4 involved in SSc using multi-omics but also an R package called RegEnrich to reveal the mechanisms of other diseases. In addition, we have revealed shared serum proteomic signatures between patients with Pso and PsA, and divergent multi-omic signatures between responders and non-responders to TNFi using machine learning techniques. All of these findings may ultimately facilitate personalized medicine, either from a better understanding of diseases or simply from providing informative drug response predictors.